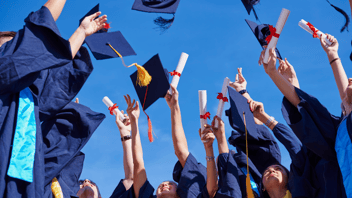
True learning analytics requires far more than data from a single platform. While assessment data—whether formative or summative—can offer critical insights, relying on it alone is akin to navigating a dark maze with just a flashlight. To illuminate the whole learner, we must weave together a tapestry of data from multiple systems, sources, and contexts. This is the foundation of what we call a "learning graph."
A learning graph is a comprehensive, multi-dimensional data structure that connects various facets of a student’s educational experience. Far from being a static repository, it dynamically integrates information from diverse sources—assessment tools, learning management systems (LMS), behavior tracking systems, attendance records, extracurricular involvement, and even socio-emotional learning (SEL) surveys. The result? A holistic, actionable picture of each student that empowers educators, administrators, and even parents to make informed decisions.
At its core, the learning graph is about solving a fundamental problem: fragmentation. Many edtech vendors focus narrowly on their own data, building the next best assessment or LMS tool. While these systems may be excellent at what they do, they often operate in silos, providing only partial insights. This approach leaves educators grappling with disconnected pieces of the puzzle and overwhelmed with too many sources of information.
For instance, a math assessment platform may show that a student struggles with fractions, but it cannot reveal whether this challenge stems from inconsistent attendance, a lack of foundational skills, or a broader disengagement with learning. To support learners holistically, we need to connect the dots across datasets and systems. This is where the learning graph becomes indispensable.
Building a learning graph requires data engineering at scale. Here are just a few of the data types that must be integrated:
Assessment Data: Performance on standardized tests, classroom quizzes, and adaptive assessments. Challenges: Data formats vary widely across platforms, requiring normalization.
Grades Data: In-progress and transcript grades. Challenges: Elementary and secondary grades can be very different, may or may not be standards-based, making automation more difficult.
Behavior and Engagement Data: Attendance, disciplinary records, and LMS engagement metrics like time on task or completion rates. Challenges: These data often lack standard definitions or consistent reporting across districts.
Socio-Emotional Learning (SEL) Data: Survey results measuring skills like self-regulation, empathy, and social awareness. Challenges: Surveys can be infrequent and subjective, necessitating careful interpretation.
Contextual Data: Demographics, English language proficiency, special education needs, and family engagement metrics. Challenges: Privacy and data security are critical concerns when working with sensitive information.
Longitudinal Data: Historical records that track a student’s progress over time, essential for identifying trends and trajectories. Challenges: Districts often have disparate systems with limited interoperability, making it hard to consolidate historical data.
The process of building a learning graph is both art and science. It requires robust data pipelines, advanced processing techniques, and a commitment to data governance. Here are the key steps:
Recent advancements in AI and machine learning (ML) have ushered in a new era for learning analytics. As highlighted in a recent EdSurge article, AI tools are increasingly dependent on large, diverse datasets to deliver meaningful insights. However, the efficacy of these tools hinges on the quality and breadth of the underlying data—and this is precisely where the learning graph comes into play.
AI models excel when they can analyze relationships and patterns across a wide array of variables. A narrowly scoped dataset—such as scores from a single assessment—lacks the contextual richness needed for advanced applications. By contrast, a learning graph provides a multidimensional dataset that fuels AI algorithms with the depth and variety they need to:
The true power of the learning graph lies in its ability to generate actionable insights. For teachers, it can highlight correlations between SEL scores and academic performance, enabling targeted interventions. For administrators, it provides a bird’s-eye view of equity gaps across schools or subgroups. For parents, it delivers a comprehensive report that contextualizes their child’s progress.
Moreover, a well-constructed learning graph serves as a springboard for generative AI applications specifically. AI models trained on rich, multi-dimensional data can:
Without a learning graph, the promise of AI in education remains constrained by data silos and limited scope. For example, a generative AI tool that creates lesson plans or recommends interventions will fall short if it cannot incorporate behavioral and SEL data alongside academic metrics. This comprehensive integration is what elevates AI from a novelty to a truly transformative tool in education.
While the potential of the learning graph is immense, the journey is not without hurdles:
For edtech vendors building a single-purpose tool, focusing on internal data might suffice. But for those aiming to support learners holistically, the learning graph is not optional—it is essential. By integrating data from multiple sources and systems, we can move beyond fragmented insights to deliver comprehensive, actionable analytics that truly empower educators, administrators, and parents.
At Schoolytics, we believe that the learning graph is the missing puzzle piece in modern education. Building it requires deep expertise in data engineering, a commitment to interoperability, and a focus on the end goal: supporting every student’s learning journey. It’s a challenging endeavor, but one that has the potential to transform education as we know it.
School districts are facing budgetary constraints that make it difficult to pay for new, innovative...
Surveys like the Panorama Student Survey provide invaluable insights into the social-emotional...